離散捲積 (Discrete Convolution)
給定兩個數列 $A = (a_0, a_1, \dots a_{n-1}),\ B = (b_0, b_1, \dots, b_{m-1})$,
求兩數列的離散捲積 $C = (c_0, c_1, \dots, c_{n+m-2})$,其中 $$c_k = \sum_{i + j = k}a_ib_j$$
我們可以將數列轉換成多項式:
$$\begin{align} A(x)=a_0+a_1x+a_2x^2+\dots+a_{n-1}x^{n-1}\\B(x)=b_0+b_1x+b_2x^2+\dots+b_{m-1}x^{m-1}\end{align}$$
這樣一來,$c_i = (A * B)(x)$ 在 $x^i$ 項的係數,如果用最 naive 的做法,總共要花 $O(n\times m)$ 的時間。
這裡的目標是要在 $O((n+m)\log (n+m))$ 的時間算出 $C$。
多項式的表示法
係數表示法 Coefficient Representation
對於一個 $n-1$ 次多項式 $F(x)=a_0+a_1x+a_2x^2+\dots+a_{n-1}x^{n-1}$,
我們可以用 Coefficient Representation 來表示他:
$F(x) := [a_0, a_1, \dots, a_{n - 1}]$
點值表示法 Point-value Representation
除此之外,令 $x_0, x_1, \dots, x_{n-1}$ 為 $n$ 個不同的數字,
我們也能用這些點在 $F$ 中的取值來表示他
令 $y_i = F(x_i),\ i = 0, 1, \dots, n-1$,
則 $F(x):= [y_0, y_1, \dots, y_{n - 1}]$
這種表示法又叫做 Point-value Representation
新的思路
給定 Coefficient Representation,我們現在只會 $O(n\times m)$ 來做多項式乘法。
那如果換成 Point-value Representation 呢?
$C(x_i) = A(x_i) \times B(x_i),~i = 0, 1, \dots, n+m-2$
我們只要能抓 $n+m-1$ 個不同數字的取值,最後一個對一個再相乘起來就好了!只需要 $O(n+m)$ 的時間。
我們可以把計算多項式乘法的任務轉換成:
- 選擇 $n+m-1$ 個不同的數字 $X=(x_0, x_1, \dots, x_{n+m-2})$
- 將原本是 Coefficient Representation 的多項式 $A,B$ 轉為 Point-value Representation
- 在 $O(n+m)$ 的時間計算 $C(x_i) = A(x_i) \times B(x_i)$,得到用 Point-value Representation 表示的多項式 $C$
- 將多項式 $C$ 轉換回 Coefficient Representation
只要好好的選擇 $X$,就可以用分治法 (divide and conquer) 加速步驟 2, 4。
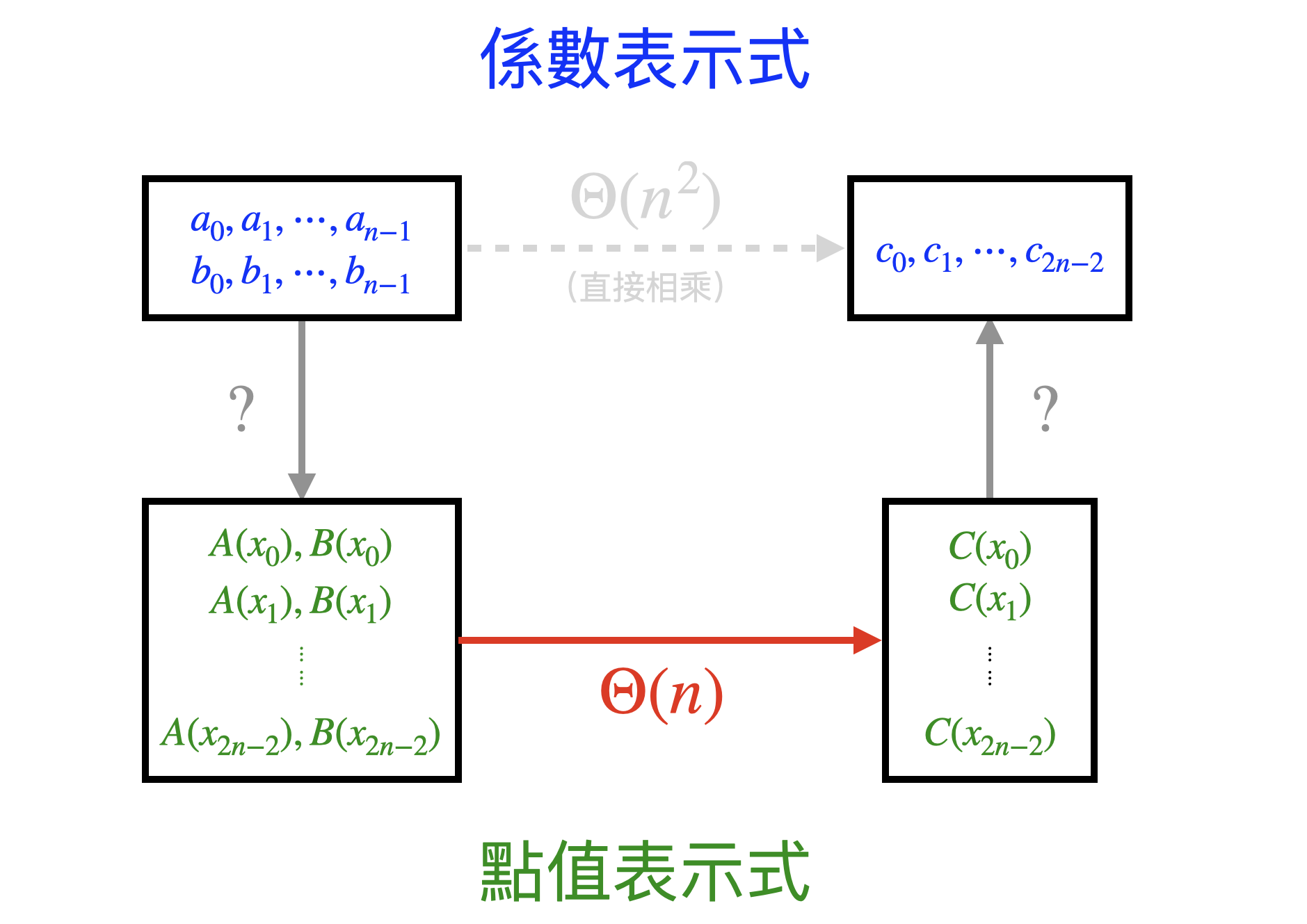
$X$ 的選擇
當 $n=2^r,r\ge 0$ 的時候,假設有個 $\omega(n)$ 函數有以下性質:
- $\omega(n)^0, \omega(n)^1,...,\omega(n)^{n-1}$ 皆為不同數值
- $\omega(n)^n=1$
- $\omega(n)^{\frac{n}{2}}=-1$,其實條件 1, 2 同時滿足的話這點會自動成立
- $\omega(n)^2=\omega(\frac{n}{2})$
設 $$X=(x_0, x_1, \dots, x_{n-1}),~x_i=\omega(n)^i$$ 則原本是 Coefficient Representation 的多項式 $$F(x) := [a_0, a_1, \dots, a_{n - 1}]$$ 其 Point-value Representation $$F(x) := [y_0, y_1, \dots, y_{n - 1}],~y_i=F(x_i)$$
透過 $\omega(n)$ 函數的性質可以利用分治法在 $O(n \log n)$ 的時間遞迴求出來。
若 $n$ 不是 2 的冪次,我們可以找到一個 $n'=2^r,n'>n$,將 $a_n,a_{n+1},...,a_{n'-1}$ 都設為 0,則 $$F'(x)=a_0+a_1x+...+a_{n'-1}x^{n'-1}$$ 就能滿足使用分治法的條件。
分治法 (divide and conquer) 求 Point-value Representation
假設有個函數 $DC(F, n)$ 輸入一個 $n-1$ 次多項式 $F(x)$ 的係數表示法,回傳 $[F(\omega(n)^0), F(\omega(n)^1),..., F(\omega(n)^{n-1})]$。
設 $$\begin{align}
G(x)=a_0+a_2x+a_4x^2+...+a_{n-2}x^{\frac{n}{2}-1}\\
H(x)=a_1+a_3x+a_5x^2+...+a_{n-1}x^{\frac{n}{2}-1}
\end{align}$$ 由 $F$ 的係數得到 $H,G$ 的係數只需要 $O(n)$ 的時間。
我們可以把 $F(x)$ 用 $G(x)$ 和 $H(x)$ 表示:
$$F(x)=G(x^2)+x\times H(x^2)$$ 透過 $DC$ 函數可以遞迴得到 $$\begin{align}
DC(H,n/2)&=[H(\omega(n/2)^0), H(\omega(n/2)^1),..., H(\omega(n/2)^{n/2-1})]\\
DC(G,n/2)&=[G(\omega(n/2)^0), G(\omega(n/2)^1),..., G(\omega(n/2)^{n/2-1})]
\end{align}$$
對於 $0\le k<\frac{n}{2}$,透過性質 2, 3, 4 可以知道:
$\begin{align}
F(\omega(n)^k)&=G(\omega(n)^{2k})+\omega(n)^k\times H(\omega(n)^{2k})\\
&=G(\omega(n/2)^k)+\omega(n)^k\times H(\omega(n/2)^k)\\
\\
F(\omega(n)^{\frac{n}{2}+k})&=G(\omega(n)^{n+2k})+\omega(n)^{\frac{n}{2}+k}\times H(\omega(n)^{n+2k})\\
&=G(\omega(n)^{2k})-\omega(n)^k\times H(\omega(n)^{2k}) \\
&=G(\omega(n/2)^k)-\omega(n)^k\times H(\omega(n/2)^k)
\end{align}$
這樣有了 $DC(H,n/2),DC(G,n/2)$ 就可以在 $O(n)$ 的時間做出 $DC(F,n)$ 的結果。得到遞迴的時間複雜度 $T(n)=O(n) + 2T(n/2) + O(n) = O(n\log n)$。
舉例來說 $n=8$
$F(x)=a_0+a_1x+a_2x^2+...+a_7x^7$
$$\begin{align}
G(x)=a_0+a_2x+a_4x^2+a_6x^3\\
H(x)=a_1+a_3x+a_5x^2+a_7x^3
\end{align}$$
想要用遞迴方法求出 $F(\omega(8)^0), F(\omega(8)^1),..., F(\omega(8)^7)$。
首先可以遞迴求出
$$\begin{align}
G(\omega(4)^0), G(\omega(4)^1), G(\omega(4)^2), G(\omega(4)^3)\\
H(\omega(4)^0), H(\omega(4)^1), H(\omega(4)^2), H(\omega(4)^3)
\end{align}$$
接著可以在 $O(n)$ 得到:
- 用加的
- $F(\omega(8)^0)=G(\omega(4)^0)+\omega(8)^0\times H(\omega(4)^0)$
- $F(\omega(8)^1)=G(\omega(4)^1)+\omega(8)^1\times H(\omega(4)^1)$
- $F(\omega(8)^2)=G(\omega(4)^2)+\omega(8)^2\times H(\omega(4)^2)$
- $F(\omega(8)^3)=G(\omega(4)^3)+\omega(8)^3\times H(\omega(4)^3)$
- 用減的
- $F(\omega(8)^4)=G(\omega(4)^0)-\omega(8)^0\times H(\omega(4)^0)$
- $F(\omega(8)^5)=G(\omega(4)^1)-\omega(8)^1\times H(\omega(4)^1)$
- $F(\omega(8)^6)=G(\omega(4)^2)-\omega(8)^2\times H(\omega(4)^2)$
- $F(\omega(8)^7)=G(\omega(4)^3)-\omega(8)^3\times H(\omega(4)^3)$
程式碼的部分等講完逆變換後在介紹。
逆變換
設 $(y_0, y_1, \dots, y_{n - 1}),~y_i=F(x_i)$,令多項式 $Z(x)=y_0+y_1x+y_2x^2+y_{n-1}x^{n-1}$,也就是將 $F(x)$ 的 Point-value Representation 作為多項式 $Z(x)$ 的 Coefficient Representation。
將 $\omega(n)^k$ 帶入 $Z(x)$ 可以發現
$$\begin{align}
Z(\omega(n)^k)&=\sum_{i=0}^{n-1} F(\omega(n)^i)\omega(n)^{ik} \\
&=\sum_{i=0}^{n-1} \left(\left(\sum_{j=0}^{n-1} a_j\omega(n)^{ij}\right)\omega(n)^{ik}\right)\\
&=\sum_{j=0}^{n-1}a_j\left(\sum_{i=0}^{n-1} \left(\omega(n)^{j+k}\right)^i\right)
\end{align}$$
這裡等比數列的和只有兩種可能
$$\sum_{i=0}^{n-1} (\omega(n)^{j+k})^i = \left\{
\begin{aligned}
&n&,&~~~j+k\equiv 0\ (mod\ n) \\
&\frac{\omega(n)^{n(j+k)}-1}{\omega(n)^{j+k} -1} = 0&, &~~~\text{else}
\end{aligned}
\right.$$
因此得到結論
- $Z(\omega(n)^0)=a_0\times n$
- $Z(\omega(n)^k)=a_{n-k}\times n, ~~~0<k<n$
這表示我們可以將 $y_0\sim y_{n-1}$ 使用同樣的分治法輕鬆地在 $O(n \log n)$ 得到原本多項式 $F(x)$ 的係數 $a_0\sim a_{n-1}$
遞迴版本程式碼
由於我們還不知道 $\omega(n)$ 究竟是個怎樣的函數,實作使用 template 的方式,使用者要將與 $\omega(n)$ 有關的操作寫成 class 後填入 `Policy` 這個欄位。
$\omega(n)$ 的選擇
可以觀察到 $\omega(n)^k$ 有非常明顯的循環性質,這在一般人常見的實數領域中很少見,有這種性質的東西經常出現在:
- 複數運算的單位根
- 同餘運算下的有限體 (finite field)
快速傅立葉變換 (Fast Fourier Transform, FFT)
設 $\omega(n)=e^{i\frac{2\pi}{n}}$。透過 Euler's formula 可以知道 $e^{i\frac{2\pi}{n}}=\cos(\frac{2\pi}{n})+i\sin(\frac{2\pi}{n})$
這樣 $\omega(n)$ 的數學含意就是複數的 $n$ 次單位根。
- $\omega(n)^0, \omega(n)^1,...,\omega(n)^{n-1}$ 的值皆不相同
- $\omega(n)^n=e^{i\times 2\pi}=1$
- $\omega(n)^{\frac{n}{2}}=e^{i\pi}=-1$
- $\omega(n)^2=e^{i\frac{2\times 2\pi}{n}}=e^{i\frac{2\pi}{n/2}}=\omega(\frac{n}{2})$
複數以及 `exp` 函數都是 C++ STL 有提供的東西:
不過使用 FFT 計算多項式乘法會產生浮點數誤差,因此有些人會考慮使用待會會介紹的 FNTT
快速數論變換 (Fast Number-Theoretic Transform, FNTT)
設 $\omega(n)=g^{\frac{P-1}{n}}\mod P$,這裡的 $P$ 是滿足某性質的質數且 $g$ 是$\mod P$ 的原根。因此首先我們要來認識什麼是原根。
什麼是原根
假設 $g, m$ 互質, 使得 $g^d \equiv 1\ (mod\ m)$ 成立的最小正整數 $d$ 定義為 $\delta_m(g)$。
根據歐拉定理 $\delta_m(g)|\phi(m)$,若 $\delta_m(g) = \phi(m)$ ,則稱 $g$ 是$\mod m$ 的原根 (primitive root)。
如果 $m$ 是個質數,則最小的 $g$ 通常是個很小的數字 ($g\ll P^{5/\log\log P}$ by Least Prime Primitive Roots),zerojudge 上剛好有一題 [b435. 尋找原根]。
對於任意質數 $P>2$ 其原根 $g$ 有一些直觀的性質:
- $\phi(P)=P-1,~g^{\phi(P)}\equiv g^{P-1}\equiv 1\ (mod\ P)$,這其實就是費馬小定理
- $g^1,...,g^{P-2},g^{P-1}$ 在$\mod P$ 的結果皆不相同,這是原根本來的性質
- $g^{(P-1)/2}\equiv -1\ (mod\ P)$ ,由性質 1,2 可以得到
如何選擇質數 $P$
若 $P-1$ 可以被 $n$ 整除,則所有 $\omega(n)$ 的性質都能滿足(所有運算皆是同餘運算):
- $\omega(n)^0, \omega(n)^1,...,\omega(n)^{n-1}$ 的值皆不相同
- $\omega(n)^n=g^{\frac{P-1}{n}n}=g^{P-1}= 1$
- $\omega(n)^{\frac{n}{2}}=g^{(P-1)/2}=-1$
- $\omega(n)^2=g^{\frac{2(P-1)}{n}}=g^{\frac{P-1}{n/2}}=\omega(\frac{n}{2})$
為了滿足 $P-1$ 可以被 $n$ 整除,因為 $n$ 是 2 的冪次,FNTT 需要一個特殊構造的質數 $P=r\times 2^k+1,~2^k\ge n$,已經有中國人整理出一些常用的質數:
$P=998244353=7\times 17\times 2^{23}+1$ 是個經常被使用的質數,其原根 $g=3$。
這樣我們就可以輕鬆地根據定義寫出 FNTT 的實作:
注意 FNTT 的所有運算皆是同餘運算,也就是說 FNTT 的計算多項式乘法的結果是原本的數字$\mod P$ 的值,因此若需要得到精確的結果需要用不同質數執行多次 FNTT 使用中國剩餘定理將結果合併。
假設有個 $n-1$ 次多項式要和一個 $m-1$ 次多項式做乘法,這兩個多項式的所有係數皆小於一個正整數 $q$。
那麼這樣任何多項式係數的範圍就是 $[0,q-1]$,係數兩兩相乘不會超過 $(q-1)^2$,一共最多 $\min(n,m)$ 項相加,不會超過 $\min(n,m)\times(q-1)^2$。
我們可以選 $k$ 個可以進行 FNTT 的不同質數使得以下條件成立:
$$\prod_{i=1}^{k}p_i>\min(n,m)\times(q-1)^2$$
這樣分別使用這些質數執行 FNTT 後再使用中國剩餘定理將結果合併就可以得到完全精確的係數,但要注意計算範圍可能會超過 `long long`,甚至有可能會需要 `__int128_t`。
非遞迴版 Cooley-Tukey Algorithm
我們將係數遞迴的狀況畫出來,注意到葉節點係數的順序會是 $(0, 4, 2, 6, 1, 5, 3, 7)$:
觀察這棵樹,由上往下的第 $i$ 次分層時,是按照其 index 在第 $i$ 個 bit 的奇偶分兩邊的,並且第 $i$ 次分層會決定其最後位子的第 $\log_2 n - i - 1$ 個 bit。
可以推論出,index $i$ 的換置後的位子就會將是 $i$ 的 binary representation 給 reverse。
Reverse Bit 的方法
遞推建表法,建立 $O(n)$ 大小表,總時間複雜度也是 $O(n)$
直接換置法,一次反轉一個數字 $n$,只要 $O(1)$ 空間,但時間複雜度是 $O(\log\log n)$
如果 index $i$ 的位置是 $j$,那麼 index $j$ 的位置也會是 $i$。
想要節省空間的話,可以考慮用直接換置法 in-place 進行換置:
蝶形網路 Butterfly Diagram
我們一開始就把係數的順序透過 bit reverse 換置,可以寫出非遞迴版本的程式碼:
將計算流程畫成圖形,可以看到有很多長得像蝴蝶的形狀,因此被稱之為蝶形網路:
離散捲積程式碼
測試程式碼
Output:
5 16 34 60 70 70 59 36
(5.0,0.0) (16.0,0.0) (34.0,0.0) (60.0,-0.0) (70.0,-0.0) (70.0,-0.0) (59.0,-0.0) (36.0,0.0)
5 16 34 60 70 70 59 36